IT Professional Curricula Enterprise Database Systems Solution Area Math The Math Behind Distance-based Models
Machine learning (ML) is widely used across all industries, meaning engineers need to be confident in using it. Pre-built libraries are available to start using ML with little knowledge. However, to get the most out of ML, it's worth taking the time to learn the math behind it.
Use this course to learn how distances are measured in ML. Investigate the types of ML problems distance-based models can solve. Examine different distance measures, such as Euclidean, Manhattan, and Cosine. Learn how the distance-based ML algorithms K Nearest Neighbors (KNN) and K-means work. Lastly, use Python libraries and various metrics to compute the distance between a pair of points.
Upon completion, you'll have a solid foundational knowledge of the mechanisms behind distance-based machine learning algorithms.
Use this course to learn how distances are measured in ML. Investigate the types of ML problems distance-based models can solve. Examine different distance measures, such as Euclidean, Manhattan, and Cosine. Learn how the distance-based ML algorithms K Nearest Neighbors (KNN) and K-means work. Lastly, use Python libraries and various metrics to compute the distance between a pair of points.
Upon completion, you'll have a solid foundational knowledge of the mechanisms behind distance-based machine learning algorithms.
Objectives |
---|
Distance-based Models: Overview of Distance-based Metrics & Algorithms
|
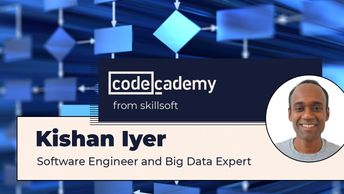