Examine fundamentals of machine learning (ML) and how Pandas ML can be used to build ML models in this 7-video course. The working of Support Vector Machines to perform classification of data are also covered. Begin by learning about different kinds of machine learning algorithms, such as regression, classification, and clustering, as well as their specific applications. Then look at the process involved in learning relationships between input and output during the training phase of ML. This leads to an introduction to Pandas ML, and the benefits of combining Pandas, scikit-learn, and XGBoost into a single library to ease the task of building and evaluating ML models. You will learn about Support Vector Machines, which are a supervised machine learning algorithm, and how they are used to find a hyperplane to divide data points into categories. Learners then study the concept of overfitting in machine learning, and the problems associated with a model overfitted to training data. and how to mitigate the issue. The course concludes with an exercise in machine learning and classification.
Objectives |
---|
Machine & Deep Learning Algorithms: Introduction
|
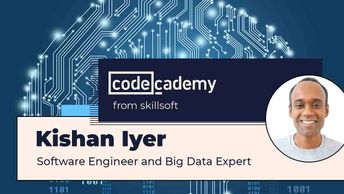